
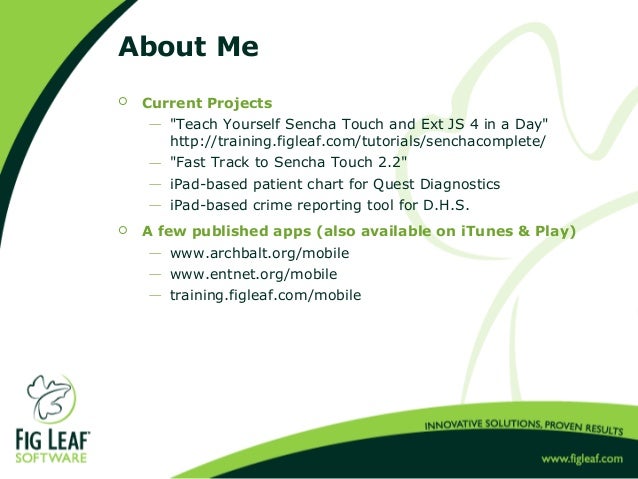
A broad debate about the ethical principles governing the development of ML-based psychiatric tools seems therefore more pressing then ever. Many further attempts to employ ML in psychiatry are under way, covering a multitude of psychiatric disorders and ranging from diagnostic and prognostic tools to the prediction of treatment outcomes. Beyond increasingly popular direct-to-consumer apps, offering for instance digital psychotherapy, the US Food and Drug Administration (FDA) recently approved the first ML-based psychiatric tool, providing diagnostic aid based on joint inputs from caregivers and attending physicians. The integration of diagnostic, predictive, and therapeutic tools based on machine learning (ML) into clinical care is accelerating-a development also apparent in psychiatry. Critical ethical research should further examine the value of explainability for an ethical development of machine learning systems and strive towards an appropriate framework to communicate ML-based medical predictions. Our findings stress the need for domain-specific ethical research, scrutinizing the use of machine learning in different medical specialties. Experts recommended increased attention to machine learning methodology, and the education of physicians as first steps towards a potential use of machine learning systems in psychiatry. Participants were divided regarding the value of explainability, as promoted by recent guidelines for ethical artificial intelligence, and highlighted that explainability may be used as an ethical fig leaf to cover shortfalls in data acquisition.

We relate these challenges to three themes, namely (1) ethical challenges of machine learning research, (2) the role of explainability in research and clinical application, and (3) the relation of patients, physicians, and machine learning system. Experts’ ethical expectations towards machine learning in psychiatry partially challenge orthodoxies from the field. We used reflexive thematic analysis to identify key ethical expectations and attitudes towards machine learning systems. We conducted semi-structured qualitative interviews with 15 experts from Germany and Switzerland with training in medicine and neuroscience on the assistive use of machine learning in psychiatry. It is crucial to accompany this development with careful ethical considerations informed by empirical research involving experts from the field, to identify existing problems, and to address them with fine-grained ethical reflection.
#Figleaf training skin#
Handle with gloves and avoid skin contact.The increasing implementation of programs supported by machine learning in medical contexts will affect psychiatry.
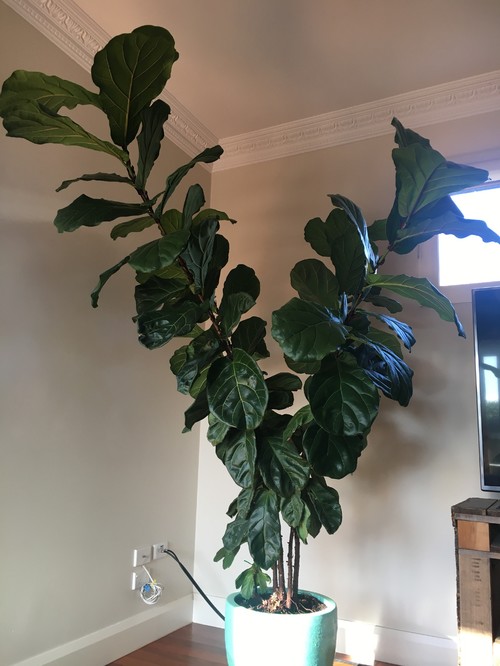
By factoring magnification, a small Figleaf can be used to give complete gonadal shielding without obscuring pelvic bone. This causes magnification of the shield shadow at the level of the ovaries and even more magnification at the film surface. When the Figleaf is positioned on the patient, the shield is separated by some distance from the ovaries and by an even greater distance from the x-ray film surface. Selection of the correct-size Figleaf is based upon the patient's abdominal thicknesses. When properly positioned, the Figleaf is secured to the skin or to close-fitting undergarments with microspore tape for best results. Proper positioning of Figleaf places the shield's vertical line directly on the mid-sagittal line of the patient's abdomen – with the bottom edge of the shield aligned 1/2" (1.3 cm) to 3/4" Unlike most shielding devices for the ovaries, uterus and fallopian tubes, our Figleaf Gonad Shields DO NOT obscure diagnostically important surrounding bone structures.įigleaf provides the protective shielding of 1mm lead equivalency and consistently blocks a minimum of 65% of all direct-beam radiation to gonadal tissues and significantly reduces scatter to vital organ areas. The Figleaf Gonad Shields effectively protect female reproductive organs during pelvic radiography, angiography and fluoroscopy. Proper shielding is vital to any woman of childbearing age and her offspring. X-Ray Markers - Radiology Markers - Lead Markers.Equipment and Patient Contact Surface Cleaning.Cassette, Imaging Plate and Screen Cleaning.
